Machine Learning Trends: Stay Ahead of the Curve
- xhqgo1
- May 14
- 4 min read
In today's fast-paced world, machine learning is transforming industries and reshaping how we interact with technology. From healthcare to finance, the impact of machine learning is profound. As we move further into the digital age, staying updated on the latest trends in machine learning is crucial for businesses and individuals alike. This blog post will explore the most significant trends in machine learning, providing insights and practical examples to help you stay ahead of the curve.
The Rise of Automated Machine Learning (AutoML)
Automated Machine Learning, or AutoML, is gaining traction as it simplifies the process of building machine learning models. Traditionally, creating a machine learning model required extensive knowledge of algorithms and coding. However, AutoML tools allow users to automate the selection, training, and tuning of models.
For example, Google Cloud AutoML enables users to train high-quality models with minimal effort. This trend is particularly beneficial for small businesses that may not have the resources to hire data scientists. By using AutoML, they can leverage machine learning without needing deep technical expertise.
Explainable AI (XAI)
As machine learning models become more complex, the need for transparency increases. Explainable AI (XAI) focuses on making machine learning models understandable to humans. This trend is essential for industries like healthcare and finance, where decisions can significantly impact lives and finances.
For instance, a healthcare provider using a machine learning model to predict patient outcomes must understand how the model arrived at its conclusions. XAI tools help demystify these models, allowing stakeholders to trust and validate the results. Companies like IBM are leading the way with their Watson AI, which emphasizes explainability in its algorithms.
Edge Computing and Machine Learning
Edge computing is another trend that is reshaping machine learning. Instead of processing data in centralized data centers, edge computing allows data to be processed closer to where it is generated. This shift reduces latency and bandwidth usage, making real-time applications more feasible.
For example, in the automotive industry, self-driving cars rely on edge computing to process data from sensors in real time. This capability is crucial for making split-second decisions on the road. Companies like NVIDIA are at the forefront of this trend, providing powerful edge computing solutions for AI applications.
Natural Language Processing (NLP) Advancements
Natural Language Processing (NLP) is a branch of machine learning that focuses on the interaction between computers and human language. Recent advancements in NLP have led to more sophisticated chatbots and virtual assistants. These tools can understand and respond to human language more effectively than ever before.
For instance, OpenAI's GPT-3 has set new standards in NLP, enabling applications that can generate human-like text. Businesses are using these advancements to enhance customer service, automate content creation, and improve user experiences. As NLP continues to evolve, its applications will only expand.
Federated Learning
Federated learning is an innovative approach that allows machine learning models to be trained across multiple devices without sharing raw data. This trend addresses privacy concerns while still enabling collaborative learning.
For example, in the healthcare sector, hospitals can train models on patient data without transferring sensitive information. This method not only protects patient privacy but also improves the model's accuracy by leveraging diverse datasets. Companies like Google are exploring federated learning to enhance their services while maintaining user privacy.
The Importance of Data Quality
As machine learning continues to evolve, the importance of data quality cannot be overstated. High-quality data is essential for training accurate models. Poor data can lead to biased or ineffective models, which can have serious consequences.
Organizations are increasingly investing in data governance and management practices to ensure data quality. This trend includes implementing data validation processes and using tools to clean and preprocess data. By prioritizing data quality, businesses can improve their machine learning outcomes and make better decisions.
Ethical Considerations in Machine Learning
As machine learning becomes more integrated into our lives, ethical considerations are gaining prominence. Issues such as bias in algorithms, data privacy, and accountability are critical discussions in the field.
For instance, biased algorithms can lead to unfair treatment in hiring processes or loan approvals. Companies are now focusing on developing ethical guidelines and frameworks to address these challenges. Organizations like the Partnership on AI are working to promote responsible AI practices and ensure that machine learning benefits everyone.
The Future of Machine Learning
Looking ahead, the future of machine learning is bright. As technology continues to advance, we can expect even more innovative applications. From personalized medicine to smart cities, the possibilities are endless.
Moreover, as machine learning becomes more accessible, we will see a surge in its adoption across various sectors. Businesses that embrace these trends will be better positioned to thrive in the digital landscape.
Final Thoughts
Staying ahead of the curve in machine learning requires continuous learning and adaptation. By understanding the latest trends, you can leverage machine learning to drive innovation and improve outcomes in your organization. Whether you are a business leader, a data scientist, or simply someone interested in technology, keeping an eye on these trends will help you navigate the ever-evolving landscape of machine learning.
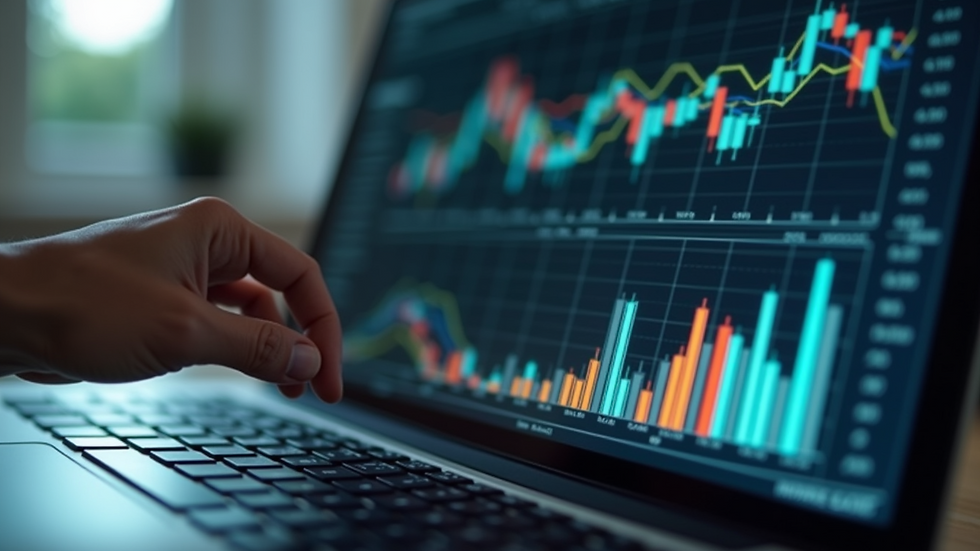
As we move forward, remember that the key to success in machine learning lies in embracing change and being open to new ideas. The future is bright for those willing to learn and adapt.
Comments